Scaling Laws of AI
Dario Amodei from Anthropic explains how increasing the size of AI models, data, and computational resources enhances performance. Scaling laws have been observed across various domains. However, potential future limits must be considered.
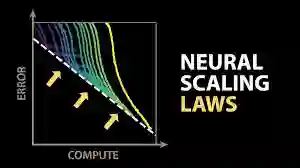
The scaling laws in artificial intelligence (AI) indicate that by increasing model size, available data, and computational resources, one achieves significant improvements in performance. This concept, supported by Dario Amodei, CEO of Anthropic, originates from his direct experience in the field, which began about ten years ago with his initial studies on speech recognition.
Amodei already observed at that time that larger models and broader datasets substantially enhanced performance quality. Initially, he believed this phenomenon was limited to specific domains like speech recognition; however, the emergence of GPT-1 in 2017 radically changed his perspective, demonstrating that language, too, could enormously benefit from similar approaches.
According to Amodei, scaling laws have now been confirmed in numerous areas beyond language, extending also to images, videos, and various other data types. The parallel proposed by Amodei is that of a chemical reaction: to achieve optimal results, all ingredients—network size, data volume, and training time—must be increased proportionally.
A central question addressed by Amodei concerns why larger models and bigger datasets lead to better results. The proposed answer is that larger neural networks are capable of capturing increasingly complex and less frequent patterns and correlations, thus enabling superior and more accurate performance.
Despite current successes, Amodei highlights possible future limitations: limited availability of new high-quality data, computational constraints, and the long-term sustainability of the scaling process. Despite these challenges, the current trajectory indicates that AI models are rapidly approaching unprecedented levels of competence. Indeed, recent models have demonstrated impressive results in complex tasks, such as programming and solving advanced scientific problems.
The ongoing evolution of AI could soon produce models capable of surpassing human capabilities in many domains, although, according to Amodei, the ultimate limits of scalability remain yet to be explored.
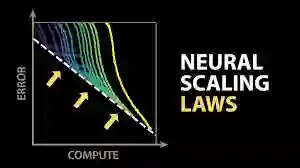
The image illustrates the concept of scaling laws applied to artificial intelligence models. The horizontal axis represents computational power ("compute"), while the vertical axis indicates the error made by models in solving a given task. The dashed white line defines the ideal achievable performance threshold—that is, the theoretical minimum limit of error. Above this line appear several colored curves, each corresponding to a specific model or configuration. These curves show that initially, as computational resources increase, performance improves significantly with a rapid reduction in error. However, beyond a certain threshold, each curve gradually slows down and tends to stabilize, indicating a maximum performance limit beyond which additional resource increases yield only minimal improvements. This implies that, to continue making significant progress, it becomes necessary to switch to larger and more complex models, enabling closer approximation to the ideal performance limit defined by the dashed line.